Safeguarding personal information is now more important than ever. 95% of customers will not engage with companies that cannot offer adequate safeguards for their data. With data protection regulations like the General Data Protection Regulation (GDPR), organizations are under constant pressure to protect sensitive data while ensuring compliance. Privacy Enhancing Technologies (PETs) have emerged as powerful tools to achieve this balance. These technologies not only help secure personal data but also support GDPR compliance by minimizing risks and enhancing confidentiality.
But what are PETs exactly, and how can they help organizations meet GDPR standards? PETs are crucial to securing data and serve a critical role PETs in modern data privacy.
What Are Privacy Enhancing Technologies (PETs)?
Privacy Enhancing Technologies (PETs) are a set of tools and techniques designed to protect personal data throughout its lifecycle. PETs can help reduce the risk to individuals while enabling further analysis of personal data without a controller necessarily sharing it, or a processor having access to it. They aim to minimize the exposure of sensitive information while still enabling data processing. PETs can be categorized based on their primary function: minimization, confidentiality, and control.
Some of the key types of PETs are as follows:
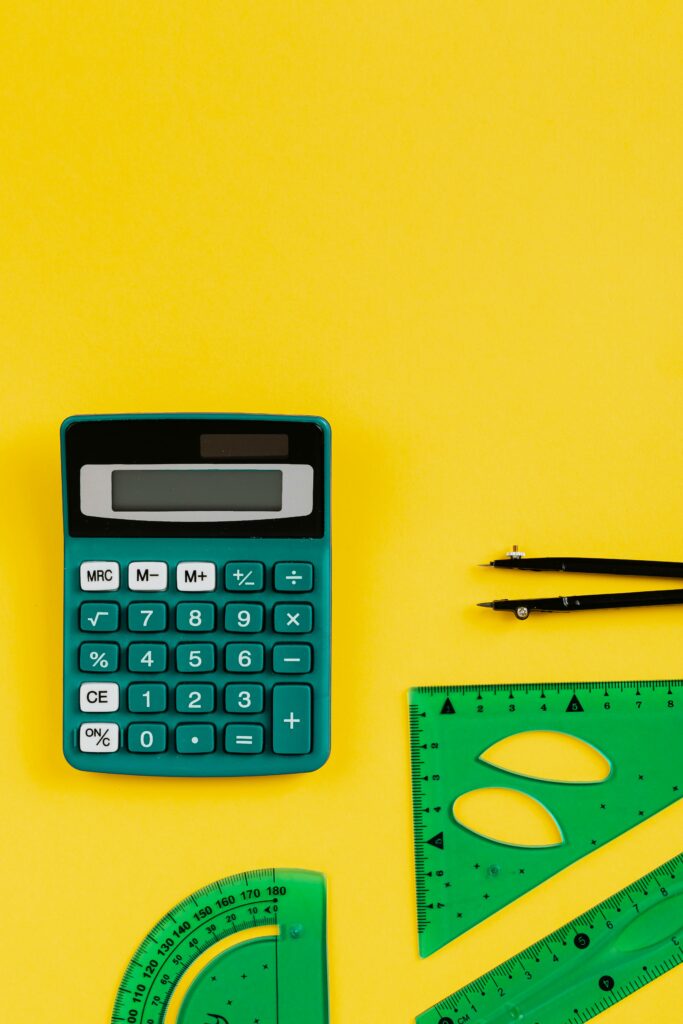
- Anonymization: This technique removes or alters personal identifiers so data cannot be traced back to an individual. Under the GDPR, true anonymization is considered irreversible; allowing the data to be stored and used without further GDPR constraints.
- Pseudonymization: Unlike anonymization, pseudonymization replaces private identifiers with artificial labels. Although it is reversible under strict controls, it adds a layer of protection by decoupling personal identifiers from the dataset. It is very important to understand pseudonymized data is not the same as anonymized data.
- Encryption: Encryption converts data into a coded format, accessible only with a specific decryption key. This ensures that even if the data is intercepted, it remains unreadable to unauthorized parties.
- Synthetic data: This allows organizations to create artificial data that mimics real data but preserves user privacy. Synthetic data is often used in AI and machine learning as well as software testing and development.
- Differential privacy: This is a mathematical concept that adds randomness or noise to data analysis, making it more difficult to identify individuals.
- Confidential computing: This form of data processing prevents unauthorized access to data during computation. It is often used in cloud computing and for healthcare and financial services.
- Federated learning: This machine learning approach allows multiple organizations to train algorithms collaboratively without sharing raw data, enhancing both privacy and compliance.
- Trusted execution environments: Secure hardware or software environments within a system that provide an isolated area of execution of sensitive operations and protect code and data from external tampering.
By using these technologies, organizations can significantly reduce the risk of data breaches and support GDPR’s core principles. PETs help to ensure that an individual’s data is better protected to avoid any potential data breaches or misuse of data.
GDPR Principles Supported by PETs
The GDPR is built around principles that prioritize data protection at every stage of processing. PETs offer a practical path to compliance by reinforcing these key principles.
The key GDPR Principles can be reinforced through the usage of PETs:
- Data Minimization (Article 5): PETs like anonymization and pseudonymization ensure that only necessary personal data is processed, reducing exposure. Techniques like differential privacy also enable organizations to analyze data sets without exposing individual identities, aligning with GDPR’s minimization principle.
- Integrity and Confidentiality (Article 5): Technologies such as encryption protect data against unauthorized access, maintaining its confidentiality and integrity. Homomorphic encryption, for instance, allows for computations on encrypted data without revealing its contents, offering enhanced protection.
- Technical and Organizational Measures (Article 25): Implementing PETs as part of system design supports privacy by design, a core requirement of the GDPR. This includes pseudonymizing or encrypting data by default, ensuring that privacy safeguards are active even before processing begins.
Organizations can further strengthen their compliance by incorporating PETs into Data Protection Impact Assessments (DPIAs), identifying and addressing potential risks before processing begins. DPIAs help document how PETs mitigate risks by offering a transparent view of data processing activities.
PETs and International Data Transfers
Cross-border data transfers are a major concern under the GDPR, especially after the Schrems II ruling. PETs help address these challenges by adding layers of security to data during transit. Technologies like encryption and federated learning ensure that sensitive information remains protected even during international exchanges. PETs act as supplementary measures to meet the GDPR Chapter 5 (Art 44-50) requirements, reducing risks during cross-border transfers and maintaining compliance with European standards.
Some examples of how PETs can help mitigate this include federated learning that allows for machine learning models to be trained across multiple locations without sharing raw data. This reduces exposure and facilitates compliance with strict European data protection laws. Encryption helps to further ensure that even if data is intercepted during transfer, it remains unreadable without the right decryption keys.
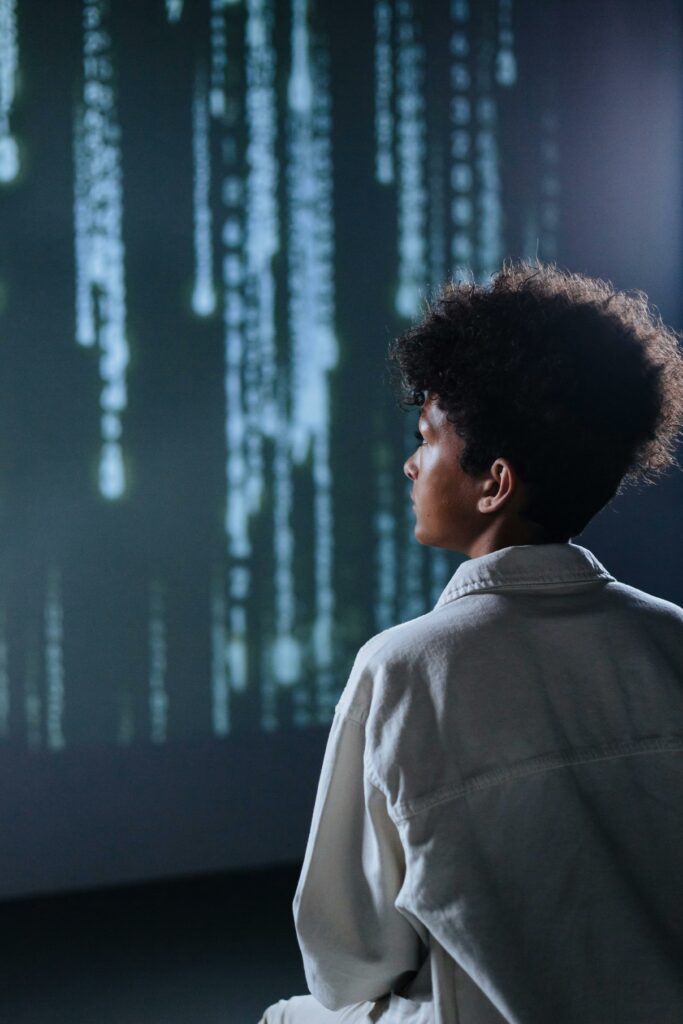
Real-World Applications of PETs
PETs are already being used across various industries to maintain privacy and GDPR compliance.
Here are some of core examples of PET usage:
- Healthcare: Differential privacy allows hospitals to share patient data for research while protecting confidentiality.
- Technology: Companies like Google and Apple use federated learning to improve their services without centralizing user data. Apple also uses differential privacy.
- Finance: Secure computation enables financial institutions to analyze sensitive data while maintaining strict confidentiality.
Implementing PETs requires careful planning and collaboration across IT, legal, and privacy teams. Legal ambiguities around anonymization, integration with legacy systems, and the complexity of deployment can pose challenges. However, conducting DPIAs, aligning strategies with GDPR Article 32, and ongoing training for staff help smooth the integration process. Regular audits and collaborative cross-functional efforts also contribute to effective implementation.
PETs as a Strategic Enabler for GDPR Compliance
Privacy Enhancing Technologies are not just compliance tools; they are strategic assets that enable secure, responsible data processing. For organizations striving to meet GDPR standards, PETs offer a practical path to data minimization, enhanced confidentiality, and secure international transfers.
Implementing PETs as part of your data privacy strategy not only reduces compliance risks but also fosters trust with clients and partners. By embracing these technologies, businesses can navigate the complexities of GDPR with confidence and accountability.